8 Marketing Data Issues That Might Be Holding You Back (and How to Fix Them)
Let’s dive into some data pitfalls that we see even large successful marketing operations struggling with.
We can split your data sources into two main types:
First-Party Data - The data tracking that you are responsible for setting up and managing within your business. It is what’s captured within your website analytics tool(s), CRM database and email marketing platform. It can also include other data you should ideally be keeping track of, such as media and social mentions, client surveys and reviews.
Third-Party Data - The data that is provided to you by advertising platforms via their own analytics tools. This typically includes channels like Facebook, Google Ads, LinkedIn, or industry news platforms.
First-Party Marketing Data Integrity

For your first-party marketing data integrity, the common pitfalls and remedial actions include:
Issue #1: Lack of a Structured Data Model
What marketing and sales tech stack do you need to track and report data at each step of your customers journey? How do those systems need to interact and share data? What processes and people do you need to fulfill that across which teams?
Without a clear and cohesive model, siloed software systems or behavior across teams will cause data integrity issues. A well-designed data system should function like a supercar, with each component fully optimized and working in harmonious synchronization.
Action: Build and Document a Model for How Data Flows Between Platforms
Build a holistic data model with your KPI reporting requirements in mind. Create a data flow diagram of each granular step from start to finish. Include technology requirements, processes, roles, and clear definitions of what data qualifies as leads or conversions, etc.
Use this as a live document and update it with any changes to your marketing operation.
Issue #2: Poor Data Governance Processes
Symptoms of weak data governance often include a lack of format standardization. Inconsistent data entry without mandatory fields. Inconsistent tracking code usage. Naming errors, or an absence of naming rules. Too many custom data fields being created. Data being spread across multiple similar fields.
These issues regularly affect form fills, email marketing, and organic content on social media or search engines. For example:
- Website form-fills aren’t using mandatory fields, and fields are inconsistently linked to corresponding CRM fields.
- UTM code naming errors result in confusion around the specific source of sales leads and conversions.
- Marketing emails are created by duplicating previous campaigns that are missing fields for UTM codes, or have copied over old codes.
- Social media posts missing UTM codes on their links.
Action: Set Uniform Naming Conventions and a Process for Data Governance
For each system, determine which data input fields are truly necessary and/or mandatory. Bear in mind that collecting more data requires more data governance, can result in information overload, and increases the potential for dirty data.
Create clear data entry processes and defined naming rules. That includes naming rules for tracking codes and data entry fields, as well as controls for creating new data entry fields.
Make the effort to ensure that data governance processes are clearly documented and communicated for specific staff roles, process documents can be easily accessed for reference, and that the rules are continually adhered to.
Data validation tools are available within databases such as Salesforce. Consistently set which data fields are mandatory, what standardized format data should be in, and set range constraints if applicable.
Use UTM tag managers such as Google Tag Manager (free) and Tag Inspector. They’ll help you to keep naming conventions consistent and avoid tag duplication. To learn more about using UTM codes, check out our article on how to set up your digital marketing analytics.
Issue #3: Customer Error, or Unwillingness to Share Data
Your customers can easily make mistakes when entering their data in form-fills. However, if you ask customers for too much personal information early in the customer journey, they’ll be more likely to provide fake information to protect their anonymity.
They’re also more likely to abandon a conversion process if data entry is too laborious. And they could also lack trust around how their data is protected and used.
Action: Build Trust and Standardize Fields
Collect data from your customer in stages with progressive profiling as the relationship builds, using demonstrated value as justification.
Pre-population of forms and being able to save details in a user account reduces the laboriousness of your customer data capture processes.
Verifying data increases the chance of converting subscribers to paying customers. Use automated data verification tools to ensure that data is correct at point of entry. For example, use captcha validation on form fills to check its a real person, and send real-time verification emails at the point of capturing an email address.
Trust can be built with transparency about why you are capturing data and how you will manage the data. Don’t forget to provide this information, which can be done via linking to a dedicated webpage with your data privacy disclaimer. Reinforce justification for sharing data by immediately providing something of value, and make it easy for customers to unsubscribe if they want to.
Respecting your customers data and communication preferences is an important part of building your brand.
Issue #4: Human Error by Staff
Avoiding all human error is just not possible. At the end of the day, we are all human! Errors can happen at any point.
It could be a spelling mistake in a manual data entry. The accidental creation of a duplicate entry. Forgetting to set up data validation rules for a form-fill entry field, not asking for complete information, or not linking the form-fill entry fields to your CRM system. Getting the naming rule slightly wrong on a UTM code.
Staff turnover can also exacerbate the volume of errors, as it naturally takes people a little time to get up to speed and learn your processes. Perhaps database processes haven’t been well communicated to new staff, or made easily accessible in documented format.
Action: Standardize Data and Reduce Friction
The important thing is to catch as many errors as possible while asking people to stick to your data entry rules every single time.
Use automation options where possible to make it easier by reducing manual entry requirements. You could invest in a data integrity tool like gatedcontent.com for easy form-fill templates with standardized entry and tagging.
Prioritize regularly checking accuracy for the data that’s most crucial to your business. Allocate a champion. Make it part of the process that secondary approval is required before launching content like a campaign email or Google advert, for example.
Issue #5: Data is Difficult to Extract for Analysis
The analysis part of the equation is what having good data integrity is all about. Being able to analyze data and use it to make informed decisions is the whole point. Clearly named fields, standardized values and complete data sets really pay dividends at this stage!
However, your data is of little use if you can’t filter, extract, analyze or understand what it’s saying. And not being able to pull quick and easy to understand reports is a common problem our clients tend to have.
People are trying to export large quantities data from separate systems into spreadsheets, data crunch, and manually build reports. It’s tedious and time-consuming to do. And with humans building it, it’s vulnerable to error.
Action: Invest in Tools that Integrate with Data Platforms
Before defining any governance processes, remember to consider how data will be technically be reported. Make data capture and storage fit to your reporting or extraction technology right from the get go.
Invest in the right tech stack and data visualization tools to help you. And importantly, also invest in your staff’s skill-set so they can use the technology, then subsequently understand what data is saying.
Third-Party Advertising Data Integrity
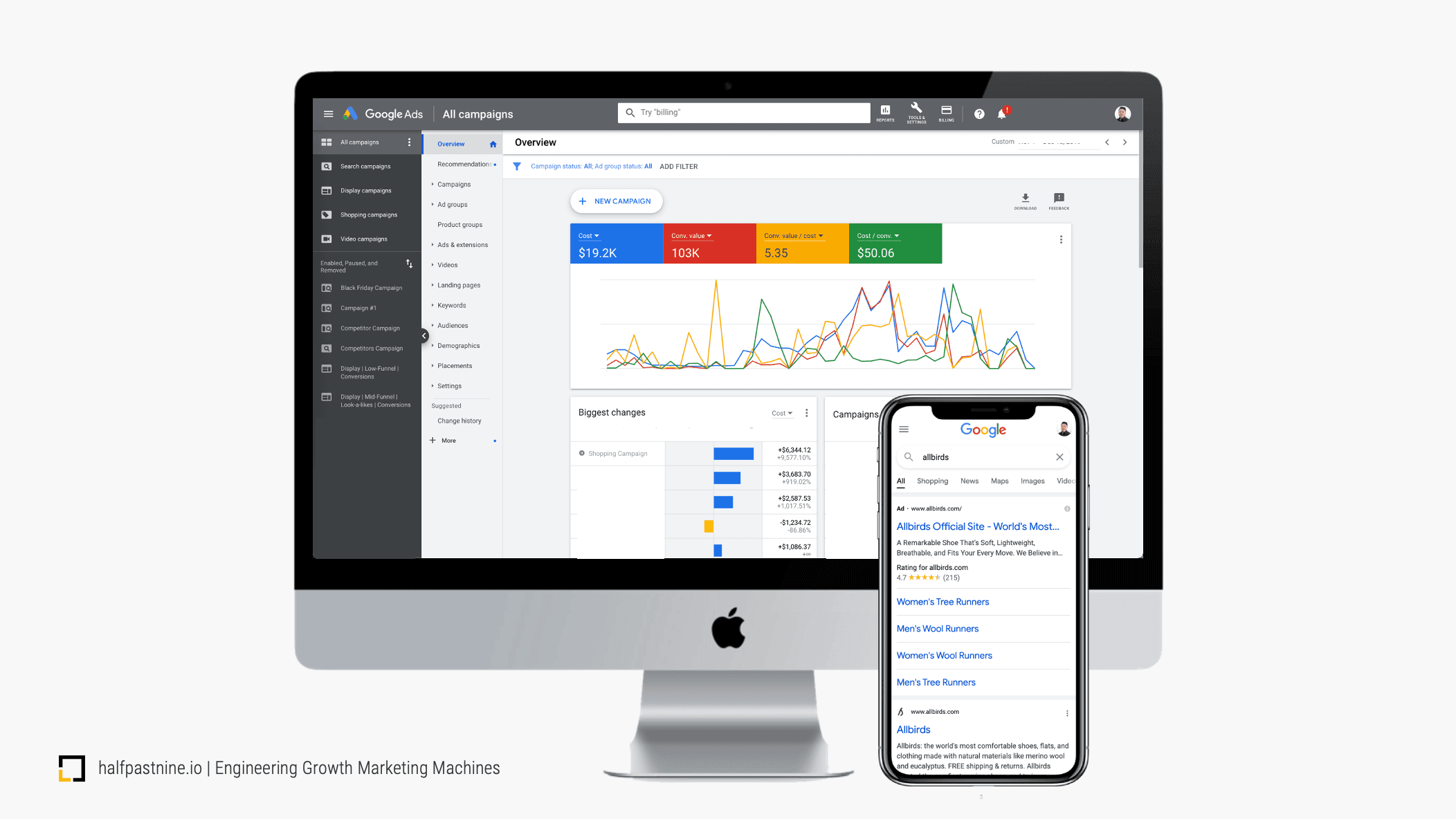
For third-party advertising data integrity, the common pitfalls and remedial actions include:
Issue #6: Not Setting Up Conversion Tracking Properly
When a new campaign is launched in paid traffic channels like Facebook Ads or Google Ads, account managers go through a data entry process that will determine what categories of information and reporting will be available for analysis after launch.
It’s your businesses responsibility to set up the pixel tracking for your adverts, and also how you define conversions so that the advertising platform can report conversions back to you. And as with in-house data, human error can come into play here also.
Without the correct set up, the in-platform reporting provided by the major digital ad providers isn’t going to provide meaningful information for you. That means you can’t use their specialized engagement reporting to asses your advert performance as a campaign progresses, or see the final Return on Ad Spend (ROAS) metrics.
Action: Check Conversion Tags
To learn more about setting up pixels and conversion tracking, check out our article on how to set up your digital marketing analytics.
Also, use tools such as Facebook’s Pixel Helper, Google Tag Assistant, and the preview function in Google Tag Manager to debug conversion tags.
Invest in leaning about the advertising platforms you are using, and make sure staff are following their best-practice guidelines. You can also require a second member of staff to provide proofing and authorization before an ad campaign is launched, ensuring processes are adhered to and human error is reduced.
Issue #7: Enhanced User Privacy Controls
Apple’s iOS 14 privacy update and the California Consumer Privacy Act (CCPA) are part of a growing trend that’s contributing to a reduction in data quality. It means that consumers can no longer be tracked from clicking an advert onto your website in order to see if they ‘convert’.
The size of the user base that these changes are impacting is significant. Thus, the accuracy of attribution and ROAS data decreases everyday.
Reporting is increasingly modeled to compensate for missing data where users couldn’t be tracked. And where all ad reporting data on key platforms like Facebook was once live, it now takes a day or two to for modeled results to be reported back for users who have opted not to be tracked. (Facebook indicates in its ad reporting where modeled data is in use.)
Action: Stay On Top of New Regulations
Sense check modeled data by monitor your ad campaigns with your own UTM tracking. You just need to insert clearly labelled UTM codes to the ad links. You’ll then be able to validate the click-through attribution data you’re given, and compensate for in-platform data that’s modeled (estimated) and delayed due to data privacy restrictions.
Note that to monitor live campaigns, you’ll need to have a good set up for quickly pulling and visualizing your UTM code data.
Issue #8: Double Attribution
Advertising attribution data is a common source of inaccurate marketing reporting. It’s also a critical one when it comes to optimizing the revenue results you get from your ad budget.
Advertising platforms are competing to demonstrate that they deliver the highest returns. They want to influence you to spend more of your ad budget with them, so they maximize the ROAS data they present. If you advertise on more than one channel at the same time, double attribution counting can easily skew your ROAS data.
How does that happen? Let’s say a customer has visited your website and purchased an item after seeing an advert on both Facebook and Google within the space of a day. They haven’t clicked on either advert, and they were actually already a customer who intended to make a purchase, which they followed through on. However, both of the advertising platforms claim 100% responsibility for the sale using View-Through attribution.
Or, perhaps a potential customer saw a Facebook advert and decided that they want to make a purchase but didn’t complete the purchase that day. However, after seeing a Google remarketing ad a couple of days later, they are reminded, and click on the advert to complete the purchase. Allocating 100% Click-Through attribution to Google for the sales conversion would be an inaccurate reflection of the user’s conversion journey.
You can see how this would make it difficult for you to accurately assess the effectiveness and ROAS of individual adverting channels or adverts, and also obscure the proportional contribution each advert has in creating a customer journey that leads to a sale.
Action: Cross Reference your In-House Data and On Platform Data Frequently
For channels such as Facebook, we advise sense checking in-platform ROAS data against revenue. Combine the ROAS results from each advertisement platform, then check if the combined revenues exceed your actual revenue in that period. You just need to multiple what you spend on an advert with the ROAS multiplier that Facebook has provided. If the combined revenue that ad platforms have reported exceeds your actual revenue, you’ll know there’s some double-attribution going on!
On Google Analytics, traffic source data from is shown on a last-click basis by default, which means the click that brought users to your site. Again, you can accurately track advert Click-Through attribution if you use UTM codes. Your website analytics platform won’t double-count users or their activity, so it’s a useful means of sense-checking the Click-Through attribution data provided to you by advertising platforms.
Finally, consider using a Media Efficiency Ratio (MER) as your north star advertising KPI metric.
Media Efficiency Ratio = Total Revenue / Total Paid Media Spend
It’s a more effective way to help you understand how your cross-channel advertising is working together to creating a customer journey with multiple touch-points. You’ll get a better sense of how well your wider ad strategy is working than when replying on just the stand-alone ROAS metrics from individual platforms, or Customer Acquisition Cost (CAC).
The Bottom Line
The value of good data compounds over time. Better data means better insights and decision making. Combined with know-how to interpret good data, the result is more effective marketing and customer communication. It will drive your brand impact and business growth for less financial investment.
Creating a holistic data capture process that maintains data integrity at each stage takes some knowledge, investment and continued prioritization. A well-designed data system should function like a supercar, with each component fully optimized and working in harmonious synchronization.
We’ve covered a lot here, and I know it can be overwhelming. If you need any support, please just get in touch with us at Half Past Nine to discuss what support you need. Data is our truly our passion, and we’d be delighted to help!